Creating “humanlike minds” is the next step in AI development
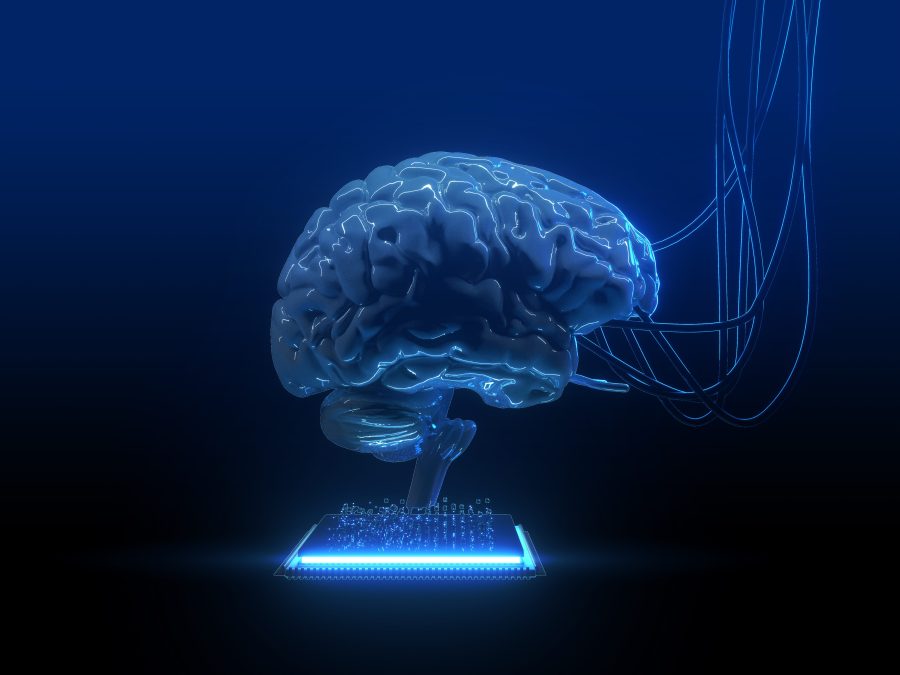
Even the most impressive artificial intelligence today isn’t quite what we see in science fiction.
The superintelligent humanoids of “Westworld,” the malevolent supercomputer in “2001: A Space Odyssey” and the emotionally attuned operating system in “Her” are all more like artificial general intelligence, rather than just artificial intelligence. They’re machines that are capable of everything humans are, or even more.
As far as we know, AGI hasn’t become a reality yet. But John Licato, a professor of computer science at the University of South Florida, told Marketplace’s Meghan McCarty Carino that experts don’t always agree on where the tipping point is.
The following is an edited transcript of their conversation.
John Licato: There’s a set of problems that we sometimes call “AI hard” or “AI complete.” And by that we mean that if any of those problems are solved by an algorithm, then we can say that that algorithm has what it takes to do everything else that a typical human can and can then be considered artificial general intelligence.
Researchers don’t all agree on which problems are AI complete, and the boundary kind of shifts. For example, 40 years ago, there were some people saying that chess is an AI complete problem. Twenty years ago, some were saying that “Jeopardy!” was an AI complete problem. Then we developed AI capable of beating the best human players in both of those games, and I think a lot of researchers would still say that AGI is something we don’t have today. So over time, we have the shifting goalposts on what the distinction is between AGI and AI and also between, the division between “human” and “artificial.”
Meghan McCarty Carino: A lot of people might have heard of the Turing test, which is this idea that the test of a machine’s ability to imitate humans is kind of the end-all, be-all of AI testing. Is that still the case, given some of the advances that we’re looking at today?
Licato: No, and actually, I don’t even think that Alan Turing, when he originally talked about this test, would have said that if we have an AI capable of passing the Turing test, then it is AGI. I think he brought it up as a, as a way of identifying a point after which the distinction isn’t going to matter that much for us. When you have an algorithm that’s able to interact with you in every meaningful way, just like in the movie “Her,” then are you really going to care as much about whether it’s real? There’s going to be a large subset of people that just don’t believe that distinction matters anymore. They’ll say, “If I can’t tell whether it’s artificial or not by interacting with it, it’s real enough for me.”
McCarty Carino: Is what we’re seeing today with AI chatbots considered artificial general intelligence? How do we know if it is or isn’t?
Licato: Unfortunately, the answer to that depends who you talk to because we still can’t come up with a consensus on what it means to be human, so we’re not going to come up with consistent answers on whether or not what we have is AGI. I think, though, that a lot of people are able to say that the reasoning used by current language models is crossing a line, and it’s reasonable to say that yes, it actually is closer to AGI than it isn’t.
McCarty Carino: There have been some claims like that among those working on today’s large language models. Researchers at Microsoft published a paper that refers to “sparks of AGI” in GPT-4 and several Google leaders were interviewed on “60 Minutes” referring to “emergent properties” in one of their language models, including translating languages they said the model had not been explicitly trained to do. These claims have been heavily criticized by some AI researchers, including Google’s own former co-lead of AI ethics, Margaret Mitchell. But, does it seem possible to you that large language models could lead to AGI?
Licato: I think the answer is a clear yes. There’s this concept in AI called the “bitter lesson” that (computer scientist) Rich Sutton talks about, and it’s the idea that in AI research, we keep coming up with these really interesting algorithms and really interesting, clever approaches to do something intelligent. And then a couple years later, we find that all we needed to do was throw more compute power at it and we would have been able to do that anyway.
That’s something that’s been happening in AI, over and over again, and the lesson is particularly bitter with language models because the underlying technology that makes state-of-the-art language models possible is actually something that we’ve had since probably the 1980s. It’s back propagation and deep networks. It’s just that we never had the available data in the sizes that we have now, and we didn’t have the compute power that we did to actually process it. Now we just threw the hardware at it, and we threw more and more money and bigger and bigger research programs at it, and it was able to get these state-of-the-art results.
So, it might be that we already have the algorithmic know-how that we need to reach AGI. It’s just that we’re waiting to have enough computational power to hit it. It could be that we’re already on that path.
McCarty Carino: We’ve been looking back at the movie “Her,” which came out 10 years ago, and at the center of that film is this relationship between a highly advanced AI operating system named Samantha, voiced by Scarlett Johansson, and a lonely guy named Theodore, played by Joaquin Phoenix. As someone who studies AI rewatching this movie now, what strikes you about Samantha, given the technology that we have today?
Licato: I was struck by the ability of the AI to sense the emotional state and emotional needs of the user and respond appropriately. There were so many times when Samantha would sense that Theodore was in a bad mood and Scarlett Johansson would just change her voice slightly so that she sounds very sympathetic and not demanding.
These are shades of what we are actually trying to do in user interface research. We’re trying to figure out how to, in some sense, anticipate what the user needs, often before they even know it themselves. Just the fact that the movie shows us a heightened version of what that could look like when we reached the goals of that kind of research is amazing to watch.
McCarty Carino: Samantha doesn’t just seem able to understand emotion, she also appears to feel emotion, or at least very convincingly give the impression that she does. Is that real? Could AGI have feelings?
Licato: I always ask my students how they know that I’m feeling emotions. I ask them, “How do you know that I’m feeling genuine emotions?” Because we just sort of infer it from a combination of little signals that we see. If you put me in a highly emotional situation, and I don’t react the way that you would expect, then you might say that I’m kind of robotic and I don’t feel emotions. But if I react in a way you expect, then you’re more likely to say, “Yeah, he’s actually feeling emotions.”
You can’t ever answer that question with 100% certainty. It’s a completely inductive inference that you make. If we have artificial intelligence systems that are so good at tailoring their outputs so that it looks like they have genuine emotional states, it’s very, very difficult, arguably impossible, to distinguish between that and whether or not they actually are feeling emotional states. At that point, you have to ask the question of whether the distinction actually matters, whether it’s actually meaningful.
McCarty Carino: If you had to guess, how far away do you think we might be from having technology like Samantha?
Licato: If I had to bet, I would say under five years. There’s a lot of possible confounding factors. There’s all this discussion of AI regulation and ethical concerns, but if the research is unconstrained and there aren’t hardware limitations, then I don’t see any reason to say that it’s more than five years away.
McCarty Carino: There’s been a lot of talk about the existential risk of developing superintelligent AI. How do you feel about the development of more advanced AI and potentially AGI?
Licato: The way I feel about it is that it’s definitely not something that we can ignore. At this point, the possibility of actually creating AGI is no longer something that we can say, “Oh, it would be interesting to deal with someday” and “Maybe some future generation will have to deal with it.” It’s very likely already here, and it’s just a matter of time before we reach it. So, we have to pay attention to it at the legislative level and at the research level.
This is arguably the most important technological development in human history. We’re essentially figuring out how to create humanlike minds. It is going to completely change the way that we interact with each other, the way societies are structured, governments, warfare, jobs — all of that is going to be completely different once we have AGI.
Related links: More insight from Meghan McCarty Carino
As John Licato mentioned, there is a fair amount of disagreement, even within the AI research community, about how close or far we are from AGI.
I mentioned Margaret Mitchell, who was the former co-lead of Google’s AI ethics team along with Timnit Gebru. Both researchers say they were fired over a controversial paper they co-authored called “On the Dangers of Stochastic Parrots.” The term “stochastic parrots” has become a skeptical way of describing large language models by critics of the technology.
We had another of the co-authors of that paper on the show this year. Emily Bender, a computational linguist at the University of Washington, told me about what she sees as the problem of “AI hype.” This was just after a number of tech leaders and researchers, including Elon Musk, signed an open letter calling for a pause in AI development based on fears that the technology would obliterate civilization.
If you want to read more of our discussion of the technology in the movie “Her,” check out Marketplace’s “Econ Extra Credit” newsletter, which comes out every Monday. “Marketplace Tech” took over the newsletter this month to examine “Her” and the ways it resonates in our current AI moment.
The future of this podcast starts with you.
Every day, the “Marketplace Tech” team demystifies the digital economy with stories that explore more than just Big Tech. We’re committed to covering topics that matter to you and the world around us, diving deep into how technology intersects with climate change, inequity, and disinformation.
As part of a nonprofit newsroom, we’re counting on listeners like you to keep this public service paywall-free and available to all.
Support “Marketplace Tech” in any amount today and become a partner in our mission.