AI is surpassing humans in several areas, Stanford report says
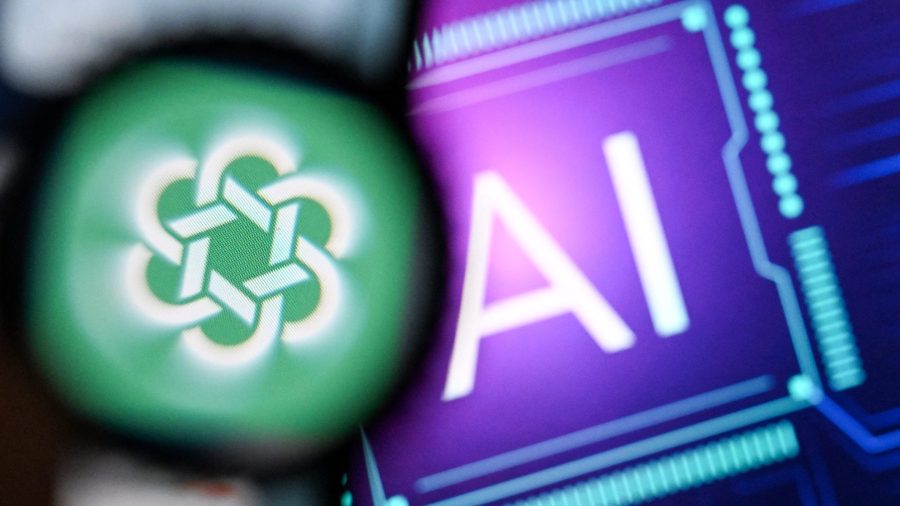
Just how capable is today’s artificial intelligence at beating humans at their own games?
That’s one of the metrics tracked by an annual report put together by the Stanford Institute for Human-Centered AI, or HAI. And its latest AI Index report finds the tech is quickly gaining on humans.
According to the report, AI now exceeds human capability not only in areas like simple reading comprehension and image classification, but also in domains that start to approach human logic, like natural language inference (the ability to draw inferences from text) or visual reasoning (the ability to deduce physical relationships between visual objects).
Still, there are areas where the bots haven’t quite caught up.
Marketplace’s Meghan McCarty Carino spoke with Nestor Maslej, research manager at HAI and editor in chief of the index report, to learn more.
The following is an edited transcript of their conversation.
Nestor Maslej: There are still some task categories, like visual common sense reasoning, which is the ability to make kind of common sense deductions from visual inputs, or competition-level mathematics, where AI trails behind humans. But even with those systems, it’s really worth noticing that the improvements in AI have been pretty remarkable in the last few years. And I think for me, it’s really not a question of if they’re going to exceed the human baseline, it’s just more a question of when. And to see how the progress has become increasingly more advanced and increasingly more rapid is really something that’s been quite interesting to behold.
Meghan McCarty Carino: There has been some speculation among AI researchers about these systems potentially hitting a wall and plateauing in terms of capabilities. Is there any evidence of that yet?
Maslej: I wouldn’t really say so. I mean, it seems like the systems are getting a lot better, and getting better a lot more quickly. And if anything, I think the question is can we keep up in terms of actually developing different evaluation suites or different means of actually tracking how well these systems have become and how strong they’ve become? One of the things that you’re really seeing in the AI landscape in the last three, four years, is — there was a significant paper that came out in, I think it was 2020, that showed that if you just basically scale these large language models or these transformer based systems — that is, you give them more data — they tend to perform a lot better on a variety of tasks. So that’s really what you’ve seen in the AI world, these major developers like Google, OpenAI and Anthropic have just really put their foot on the gas pedal of trying to make these systems increasingly bigger and increasingly more capable. And so far, that’s yielded a lot of positive returns. Now, I think there’s a question to be asked: Are we ever going to start seeing limits to those returns? And I think at the moment, the data suggests that we still have room to go, we still have room to make improvements. How much more room? That’s definitely an open question.
McCarty Carino: Another issue that has come up, sort of anecdotally and in the literature, is the issue of models like GPT-4 degrading over time, you know, the outputs getting worse. Is that anything that you’ve looked at?
Maslej: Yeah, there is some research that we profile in the report. I think there was one study that tried to look at changes in the performance of large language models over time. And the study found that yeah, across several different kinds of tasks categories, the performance of ChatGPT and GPT-4 from March 2023 to June 2023 differed fairly substantially. And I think that in some cases, they were declines, and in other cases, they were improvements. Now, how you use them and how you think about using them, you need to be careful, because what you got maybe a few months ago isn’t necessarily what you’re getting today. I think there’s also research we profiled that talks about the question of training models on “synthetic data.” So I think one of the concerns is that maybe we might run out of data at some point in the future. And one of the things that has been suggested as a means of potentially alleviating this concern is to train models on synthetic data, that is, output that is generated by AI models. And the research so far suggests that those kinds of synthetic generations, as in models that are trained on synthetic data, don’t really perform that well on a variety of tasks, and their performance actually degrades. So whether we can reliably use synthetic data to continue training AI systems is going to become an increasingly important question in the next few years.
McCarty Carino: At a certain point, you know, do we need new benchmarks for how we judge AI advances as it becomes increasingly advanced?
Maslej: Yeah, I definitely think so. I mean, I think that’s one of the things you’ve really seen in the last few years in the AI ecosystem, the fact that there’s an increasing number of AI researchers that are pumping out new benchmarks and new modes of evaluating a lot of these AI systems. And you’ve seen a lot of new benchmarks introduced in the last few years, whether it’s HELM [Holistic Evaluation of Language Models], BIG-bench, and, I mean, you’re probably going to continue seeing more. And that’s just because the technology is evolving so quickly. For me, it’s also not just a matter of new intellectual tests, but tests that maybe really push the frontier of what AI is capable of doing. I think a lot of the benchmarks that historically have existed have been quite narrow in focus, in that they look at the performance of AI systems on a variety of narrow tasks. Like, can you classify text? Can you code? I think it’s going to become increasingly interesting to me to see how well AI systems can think flexibly and think more like humans. It’s hard for me to even know what a benchmark for something like that would actually look like. But I think broadening our horizons of what we want to do when it comes to benchmarks is going to be very important and really necessary if, as a society, we want to understand how these tools operate and how they behave.
Meanwhile, on the “AI getting good at something we didn’t intend it to get good at” side of things, researchers at the University of Illinois Urbana-Champaign recently found that GPT-4 was quite capable of writing malicious scripts that could exploit security vulnerabilities — in other words, hacking — based on feeding it publicly available data.
According to Axios, “OpenAI asked the researchers to not disclose the specific prompts they used to keep bad actors from replicating their experiment.”
And speaking of ChatGPT, it looks like its owner, OpenAI, has found a new data partner in the Financial Times. Earlier this week, the FT announced it will be partnering with OpenAI to license its content for development of the company’s AI tools.
OpenAI already has other deals with Politico, Business Insider, even the Associated Press.
But other news organizations, like The New York Times, The Intercept, and most recently, the Chicago Tribune, are suing OpenAI and Microsoft for allegedly misusing their work as training data.
The future of this podcast starts with you.
Every day, the “Marketplace Tech” team demystifies the digital economy with stories that explore more than just Big Tech. We’re committed to covering topics that matter to you and the world around us, diving deep into how technology intersects with climate change, inequity, and disinformation.
As part of a nonprofit newsroom, we’re counting on listeners like you to keep this public service paywall-free and available to all.
Support “Marketplace Tech” in any amount today and become a partner in our mission.