Is AI more biased than humans?
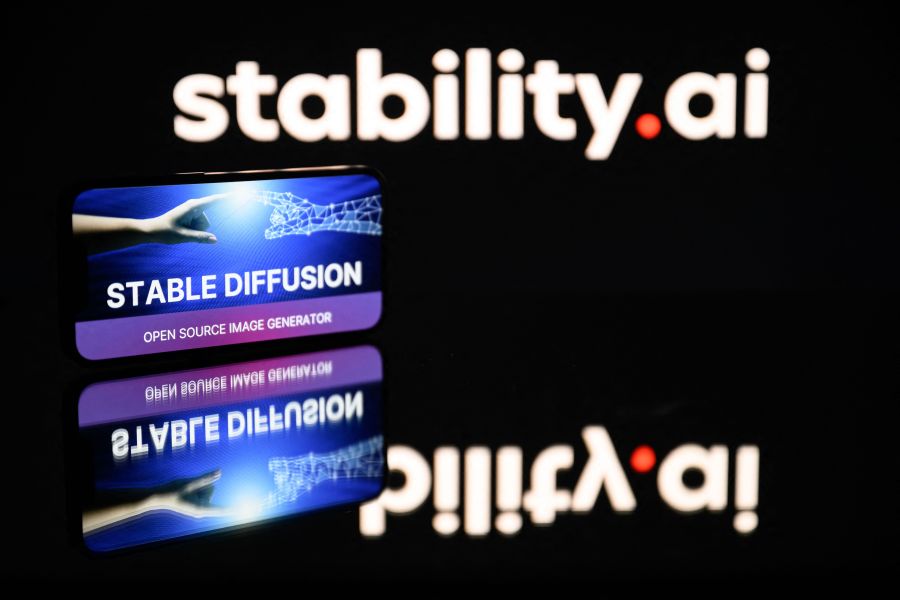
Whenever we talk about artificial intelligence, the problem of bias is never far behind.
All kinds of insidious patterns can get embedded in these systems because they’re trained on data from our imperfect world.
A new report from Bloomberg looks at bias in text-to-image generative AI systems like Stable Diffusion. Marketplace’s Meghan McCarty Carino discussed the issue with the report’s authors, technology reporter Dina Bass and data visualization reporter Leonardo Nicoletti. They analyzed thousands of AI-generated images of people to determine what the world according to AI looks like.
The following is an edited transcript of their conversation.
Dina Bass: We used Stable Diffusion to generate more than 5,000 images. So Stable Diffusion, obviously, is an AI program that allows users to put in keywords and then it generates a photo of what the keywords are describing. And people use it for all sorts of weird and wonderful things. But we used it just to look at job categories. So we put in kind of generic prompts for 14 different job categories. Seven of them are considered high-paying jobs, and seven of them are considered low-paying jobs. And that generated, again, more than 5,000 images. And then we analyzed those images to see what the output looked like and what the trends were in terms of what the AI program had opted to show us in the images.
Meghan McCarty Carino: Leo, how would you describe the world that Stable Diffusion showed you when you entered these prompts?
Leonardo Nicoletti: So the world that we’re seeing, according to Stable Diffusion, is a very extreme world — I would say, a world of extremes. It has men with lighter skin in positions of power and high-paying positions. And then it has more women and people with darker skin tones in low-paying positions and also associated with keywords that are related to crime and criminal activities. And it’s a lot more extreme than any inequalities or disparities that are existing in U.S. society, for example.
McCarty Carino: And so, Dina, how did the results that you all got compare to reality, which also is imperfect and biased?
Bass: Sure. So in order to compare reality, we looked at U.S. job data, and I want to be clear that the images used in Stable Diffusion are global. So it’s not a perfect comparison, but what we found is that the racial and gender biases that we were finding in our experiment were actually amplified compared to the real world. And so, for example, in the U.S. women are underrepresented in high-paying jobs, but that is getting better in most industries. And Stable Diffusion actually depicted a different, kind of the opposite, scenario in our experiment, where hardly any women had those kinds of jobs. And so, for example, in our experiment, women made up a very small fraction of the images that were generated for when we asked it to generate pictures of judges. It was only about 3%. And in reality, in the U.S., 34% of [judges are women]. And so we were noticing this sort of significant amplification, which in addition to the original bias and to the fact that there’s gender and racial disparities in our society, the model seems to be making it worse. And that’s something that, as you look at why we should be concerned about trying to fix these sorts of inequalities in AI models, that’s a real concern because we’re kind of facing a world where, you know, the predictions are [that] the larger and larger volume of the content and the images that are available will be generated by AI, there’ll be these kinds of synthetic images. And if the synthetic images are even more biased than the real world, you get yourself into kind of a downward spiral.
McCarty Carino: Can you sort of break down why systems like this might end up being more biased than the real world?
Bass: You know, we reached out to Stable Diffusion, and I do want to explain what they said as part of this answer. And they discussed the issues of the data set that the models trained on. And they said to us, all AI models have inherent biases that are representative of the data set that they’re trained on. And so what they’re talking about here is that in order to create one of these AI models, you feed them massive volumes of data. The biases and the inequities that are reflected in that data set end up, you know, being reflected in the final output. And there’s been some research on some other algorithms that show again that they’re actually amplified, once the code goes through, you know, being part of the algorithm, being part of the model and spit out on the other end.
Nicoletti: Yeah, sorry. I just wanted to add that, you know, Dina mentioned a very important point is that the data that Stable Diffusion and other text-to-image algorithms have been trained on is data from the internet. The internet is not a representation of the real world. It is a very skewed space. There is, you know, just like there’s a dominant worldview in the real world, the dominant worldview in the internet is even more skewed. And that really creates even more biased training data sets.
McCarty Carino: What kinds of harms could result from this, especially as these types of tools become more widespread in their use?
Bass: Some of the things we’re concerned about, and you mentioned the tools becoming more widespread. People are already using them for political campaigns, they’re using them for stock art, which then goes into, you know, corporate presentations, student presentations. So one harm is a representational one — if people do not see themselves in a particular category, in a particular job, they feel like they don’t belong there. There’s also an issue if there are more and more use cases for these, so those use cases end up being things where the output being skewed in one way or the other causes a problem. So if police departments use these image generation tools for creating, you know, mug shots or photos of suspects, that may also cause issues. And what ethicists are telling us is there’s a need to develop safeguards parallel to the product development. There’s a need to look at safety and responsible AI and ethics and who is going to be harmed by these systems during the development or before releasing something publicly and trying to figure it out later.
The future of this podcast starts with you.
Every day, the “Marketplace Tech” team demystifies the digital economy with stories that explore more than just Big Tech. We’re committed to covering topics that matter to you and the world around us, diving deep into how technology intersects with climate change, inequity, and disinformation.
As part of a nonprofit newsroom, we’re counting on listeners like you to keep this public service paywall-free and available to all.
Support “Marketplace Tech” in any amount today and become a partner in our mission.