Teaching AI to think like a human
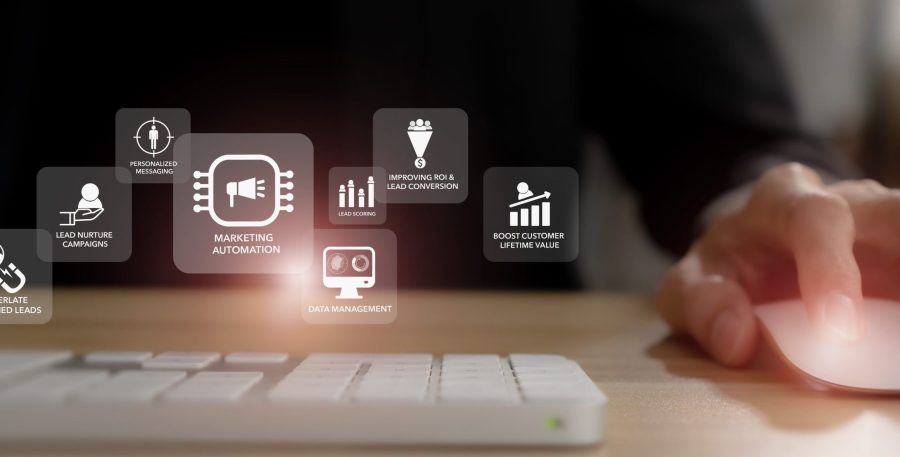
Behind the artificial intelligence tools that have become household names is an army of human workers teaching the bots to be smart.
These aren’t the folks who testify before Congress or hype the latest updates on social media. For the most part, they’re gig workers spread across the globe who do seemingly random tasks for subcontractors of subcontractors to the big-name companies that make the news.
Marketplace’s Meghan McCarty Carino spoke with features writer Josh Dzieza, who went inside the world of “data annotation” for this week’s New York magazine cover story in collaboration with The Verge.
He said the people doing this work often are given little information about who or what it’s for.
The following is an edited transcript of their conversation.
Josh Dzieza: They know that they work on a platform, and they know they’re working for companies that are training AI. But it’s often obscured through kind of inscrutable code names like “Pillbox bratwurst” or something like [that]. You can learn nothing about what you’re working on from these names. And the tasks are so small that you, in a lot of cases, can’t figure out sort of what you’re doing or what you’re training AI to do beyond, like, recognize clothing or something like that.
Meghan McCarty Carino: And you write that this tangled supply chain is sort of deliberately hard to map. I mean, you spoke with data annotators in different countries. This is kind of an ephemeral and very dynamic network that is very hard to untangle.
Dzieza: Yeah, if you think about it from the company’s perspective, you know, looking at the annotation they’re requesting, it reveals a lot about what they’re working on and how they’re training their AI. But you also need thousands and thousands of people to work on it, often for low pay. And so it’s hard to control leaks. And so one way of doing that is you tell the workers that they’re not supposed to talk to anybody, even their fellow co-workers, about it. But then you also just kind of layer these sort of various layers of anonymity over the work. And so they couldn’t really tell you much about what they were working on, even if they wanted to.
McCarty Carino: And how much does this work pay?
Dzieza: It varies a lot. So I talked to annotators all over the world. The annotators in Kenya that I spoke with, they were making a dollar or two an hour. I also spoke with people in the U.S. who were doing predominantly kind of domain-specific language stuff, like rating chatbot responses, or even doing something more specific like programming. And they were making $15 to $30 or more an hour. So there’s a lot of variability. Companies say that they try to adjust it for the local cost of living, but there’s not a lot of transparency into how that maps out.
McCarty Carino: You actually signed up for one of these tasking services where you get these gigs. Tell me about your experience.
Dzieza: It’s extremely difficult. I tried some annotation myself, and I failed at it pretty completely. One of the things that I’ve discovered is, you see sort of the top line of a task, and it’s something like “Label clothing,” and you think it’s self-explanatory, but it’s not. You know, the full instruction manual for that task was over 40 pages long, printed out. These systems are so literal and brittle that even something as simple as teaching it to recognize a shirt has “edge cases” where you only want to label shirts that are on people, but then also include mannequins, but not folded up or folded up in certain circumstances. And also, is chain mail a shirt? Is, like, a Halloween costume a shirt? You start having these edge cases pop up that the annotators encounter and the instructions get added. And pretty soon, you have this thing that’s, like, an endless list of these things.
McCarty Carino: Right. So it’s, to teach a bot sort of how to think like a human, a human has to sort of think like a bot — and kind of do these very convoluted problem-solving exercises to try to understand, like, how to get a bot to understand what a piece of clothing is?
Dzieza: Exactly. Yeah, several people described it as thinking like a robot, which is ironic, because your sole qualification for the job is often just being a human. But you have to think like a robot to classify the world in a way that you can teach robots to be human. I think one example with the clothing thing that stood out to me was, what a problem mirrors were. You know, I was told to label clothing, and there would be people taking mirror selfies, and I would label the clothing the person was wearing. But as a human, I know that the reflection of the clothing is not real clothing someone could wear. Of course, to the AI, it’s just pixels, and so you have to label the reflected clothing as well and, you know, clothing that’s in photographs in magazines, if they’re captured in the image. Sort of this extremely literal lens to view the world through.
McCarty Carino: Now at this point, there’s obviously a lot of roles and tasks for humans in this process, but there is a push to automate that work too, right?
Dzieza: Yeah, there is, and this data is expensive, so there is a lot of work happening to automate it. I think, historically, annotation work is always getting automated, but it just increases demand for new and more sophisticated types of annotation. Like you think about image recognition, and image recognition systems have no problem with the types of annotation they were trained on, kind of recognizing cats and dogs, but they enabled self-driving cars. And you need thousands and thousands or millions of people to kind of do even more detailed, even more complicated versions of image recognition. So I think there’s a world in which AI improves, it’s out in the world more, it’s encountering more edge cases and you actually need even more people doing this work.
McCarty Carino: So in general, how did you come away from this reporting feeling about how this technology is transforming work?
Dzieza: I had a lot of conflicted feelings about it. A lot of the people I talked to, you know, they were quite frustrated, especially with the precarity of it, just the cadence of AI development where you need a ton of data to train your model, and then you don’t for a bit. [That] meant they were constantly without work or doing crazy overtime because work was available and they didn’t know when it would end. And it seems to put a lot of pressure on annotators. And that was a systemic issue across kind of every form of annotation that I saw, which if this is going to be a significant part of the kind of AI economy, is this something that would need to be addressed? In terms of the work itself, I personally found it quite boring and repetitive. A lot of the people I spoke to did as well, but there were sort of higher-level jobs where, you know, you’re teaching poetry to AI and people were enjoying it and find it supersatisfying, but there were worse jobs out there. And the question was kind of how long would it last and what would it pay?
Related links: More insight from Meghan McCarty Carino
The market for human feedback and annotation in machine learning has a lot of overlap with the global outsourcing of content moderation for social media.
It’s something we talked about with Sarah Roberts of the University of California, Los Angeles, earlier this year. She’s the author of “Behind the Screen: Content Moderation in the Shadows of Social Media.”
Posts on social media platforms, generative artificial intelligence tools like chatbots and image generators need to be checked to make sure they’re not ingesting or spitting out offensive or abusive content.
So these gig workers are often serving as human filters for some of the worst material imaginable. It can be traumatizing, as Time magazine’s Billy Perrigo reported, and in places like Kenya, it pays as little as $2 an hour.
Recently, more than 150 current and former workers for various outsourcing firms in Nairobi who’ve been annotating data and moderating content for products like ChatGPT, Facebook and TikTok voted to unionize in what they’re calling the African Content Moderators Union.
The future of this podcast starts with you.
Every day, the “Marketplace Tech” team demystifies the digital economy with stories that explore more than just Big Tech. We’re committed to covering topics that matter to you and the world around us, diving deep into how technology intersects with climate change, inequity, and disinformation.
As part of a nonprofit newsroom, we’re counting on listeners like you to keep this public service paywall-free and available to all.
Support “Marketplace Tech” in any amount today and become a partner in our mission.